-
- News
- Books
Featured Books
- smt007 Magazine
Latest Issues
Current IssueTechnical Resources
Key industry organizations–all with knowledge sharing as a part of their mission–share their technical repositories in this issue of SMT007 Magazine. Where can you find information critical to your work? Odds are, right here.
The Path Ahead
What are you paying the most attention to as we enter 2025? Find out what we learned when we asked that question. Join us as we explore five main themes in the new year.
Soldering Technologies
Soldering is the heartbeat of assembly, and new developments are taking place to match the rest of the innovation in electronics. There are tried-and-true technologies for soldering. But new challenges in packaging, materials, and sustainability may be putting this key step in flux.
- Articles
- Columns
Search Console
- Links
- Media kit
||| MENU - smt007 Magazine
Estimated reading time: 7 minutes
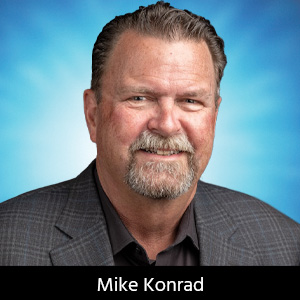
The Knowledge Base: AI in the Electronics Manufacturing Space
One of the latest acronyms to join the pop culture lexicon is artificial intelligence (AI). To many, AI brings the promise of revolutionary innovation into a near-limitless array of products and applications. To some, Stanley Kubrick’s “2001: A Space Odyssey” comes to mind, particularly the scene where the ship’s computer named HAL 9000 refuses an order from astronaut Dave to open the pod-bay doors. HAL states, “I’m sorry Dave, I’m afraid I can’t do that.” HAL followed a long line of Hollywood interpretations of artificial intelligence, frequently represented by nefarious, overzealous computers.
Like the internet itself, artificial intelligence can be harnessed for good and bad purposes. For this conversation, I’ll leave the bad purposes in the scripts of Hollywood movies.
We’ve heard the term, but what is artificial intelligence? What are its potential applications within the electronics manufacturing industry? How is it being implemented? I invited Arif Virani, co-founder and COO of DarwinAI, to explain artificial intelligence and its current and potential applications within the electronics manufacturing space.
The term “AI” has been exploited by the marketing departments of companies from almost every industry around the world. Let’s separate the wheat from the chaff. From a 30,000-foot perspective, what is artificial intelligence?
Arif Virani: You’re right, Mike. Artificial intelligence is clearly a buzzword. We think of it as the ability of machines to perform functions and tasks typically associated with humans, usually in a narrow domain. While AI can be applied broadly, the nature of the AI (e.g., expert systems, machine learning, generative AI), and the depth at which it was created, impact its usefulness.
For example, many AI packages are available to build visual inspection tools. However, getting these packages to work well in a production environment is difficult without extensive data, configuration, and deep technical know-how.
Let’s get into our way-back machine for a moment. Technologies evolve over time and AI is no exception. Explain the evolution of artificial intelligence and how different generations of artificial intelligence have evolved over time.
Virani: Expert systems have been around since the late 1950s. A human programmer would code their expertise or that of a human expert into a system. It typically translates into logical statements (e.g., “if-then-else”) representing specific rules in the decision-making process. However, it's hard for humans to explain their reasoning for a decision, making defining concrete rules extremely challenging. Furthermore, predicting every variation at the time of programming is impossible. As such, expert systems are inflexible and prone to error in real-world deployment, as they cannot handle the variations and uncertainty that can happen in practice.
Rather than hand-crafting rules as in expert systems, machine learning teaches a system how to do a task by “training” it on a large data set. The extensive rules are devised implicitly around the data. In some ways, it mimics human learning. When my daughter was a toddler, she learned what a dog was by seeing them in parks and books. When she went out with us, she could quickly point out dogs quite accurately, despite not being able to explain her rationale in expert detail. Generative AI is a form of artificial intelligence where systems create new content, such as written articles, dialogues, and imagery. Typically, generative AI is trained on past content and can generate new content based on prompts (e.g., “Generate a picture of a dog running in the field on a warm, sunny day”).
Within the electronics manufacturing space specifically, how has AI been integrated into our industry?
Virani: Here are a few examples:
- Automated Inspection: Traditional AOIs based on expert systems have been effectively used for many years. They require significant programming upfront and re-programming whenever there are changes to products and components used, changes to the manufacturing environment and processes, etc. Machine learning not only leads to a big leap in the performance of finding defects in PCB assembly but significantly reduces and even eliminates programming time and frequency.
- Design process: To help address complex designs and a scarcity of skilled engineers, software companies are adding machine learning and generative AI capabilities to automate aspects of PCBA design to speed up design and improve the efficiency of the resulting PCBA design.
- Predictive maintenance: Analytics to predict when maintenance is needed for equipment, such as a reflow oven or a pick-and-place machine, to prevent it from breaking down.
- Production analytics: Many CMs and OEMs use tools to analyze production (throughput, asset utilization, line utilization) and understand causes of downtime, maximizing production yield while at the same time minimizing waste and human labor due to rework.
You come from the inspection side of the electronics manufacturing industry. How has the integration of artificial intelligence been implemented into inspection systems and what benefits have resulted from this integration?
Virani: AI—specifically machine learning—significantly reduces programming time for inspection systems while improving inspection performance. We’ve seen programming times for inspection of a new product reduced from hours to days in a traditional AOI (e.g., Expert System) to five to 10 minutes.
Inspection performance improved, especially for areas that are difficult to address through traditional computer vision, like through-hole components, wires, FOD, and visual cosmetic inspection.
When comparing inspection systems without AI to those with AI capabilities, has the integration of AI into inspection systems expanded their capabilities, and if so, what are the added capabilities?
Virani: Systems with machine learning-based AI capabilities are more adaptable, perform better on various inspection tasks, and can provide better end-to-end analytics. Machine learning-based AI systems are more adaptable than traditional AOIs. They maintain system performance as new products come into production and alternative parts are used. For example, because a machine learning system can generally learn the difference between components such as resistors and capacitors, it is better able to handle components from new suppliers without additional programming. Furthermore, it can learn to adapt and improve its performance over time as it sees more data.
Many defect types are challenging to inspect with traditional inspection systems. As a result, instead of using such systems, given the variability seen, humans often inspect foreign object debris and damage and cosmetic defects, such as scratches and dents. A machine learning-based system can handle such variability by learning the subtleties of these challenging defects directly from data. For example, machine learning-based systems can effectively distinguish between a scratch and a cleanable mark on a box build, which would be impossible to define the rules to accomplish reliably in practice.
Newer AI inspection systems often generate large amounts of meta-data—descriptive data about an inspection. Examples include the location of the inspection in the production line, component designators, the board serial number, when the defect happened, etc. The systems can ingest additional data from other places in production, such as ICT, reflow oven, and results from other inspection systems, and use AI to help narrow down why a defect is happening and how to fix it.
Many manufacturers are emphasizing process optimization as a method for becoming more competitive. How has artificial intelligence impacted optimization on a manufacturing floor?
Virani: In high-mix, low-volume manufacturing, fast setup and turnaround time are crucial to increase throughput for EMS companies. However, inspection equipment can often become a bottleneck at many inspection points, so having a highly adaptive system that can meet dynamic manufacturing environments is key.
Moreover, automating non-value-added labor can provide immediate value by reducing labor costs, increasing throughput, and ensuring higher or more consistent quality. By implementing these strategies, manufacturers can improve their overall efficiency and profitability.
How has machine learning, and generative AI specifically, impacted the manufacturing process?
Virani: Traditionally, machine learning requires large sets of real-world data for training. With generative AI, we can work with less in environments of limited production data; we can generate more “synthetic” data to teach and improve inspection capabilities quickly.
Many manufacturing processes are optimized and produce very few defects. Using generative AI, we create images of all types of variants of defects. These are then input into our ML training to improve inspection performance.
What are the most common misconceptions of artificial intelligence?
Virani: Newer artificial intelligence is much more difficult to operationalize than people think. While it’s easy to get a simple proof of concept that will show the potential of AI, the path to production is fraught with challenges. For example, capturing images while accounting for variations in production facilities and manufacturing processes is not trivial. The adage, “garbage in, garbage out” is at play here as ML algorithms, despite their resilience, cannot function well with poor inputs.
From your perspective, where do you see the future of artificial intelligence?
Virani: As an optimist, I have a positive outlook on the future and believe that AI-based systems will work alongside humans to improve production. Although some jobs may change and certain tasks will be automated, new job opportunities will be created.
In our industry, AI will help reduce inspection costs, making it possible to have inspection capabilities at every stage of production, and potentially identifying defects as soon as they occur. This early detection will prevent further problems in the production process. By automating certain inspection tasks, operators can manage more production lines or focus on other tasks, allowing companies to increase production faster and meet growing demands.
Thank you Arif.
Virani: Happy to help.
Mike Konrad is vice president of communications for SMTA.
More Columns from The Knowledge Base
The Knowledge Base: My 2025 Industry Wish ListThe Knowledge Base: The Era of Advanced Packaging
The Knowledge Base: The Impact of Harsh Environments on Residue Tolerance
The Knowledge Base: The Pivotal Role of Solder Paste
The Knowledge Base: Enhancing Customer Loyalty and Product Quality
The Knowledge Base: The Value of Industry Certifications
The Knowledge Base: The Difference Between Critical and Distracting Decision-making
The Knowledge Base: The Transformative Role of AI and ML