-
- News
- Books
Featured Books
- smt007 Magazine
Latest Issues
Current IssueWhat's Your Sweet Spot?
Are you in a niche that’s growing or shrinking? Is it time to reassess and refocus? We spotlight companies thriving by redefining or reinforcing their niche. What are their insights?
Moving Forward With Confidence
In this issue, we focus on sales and quoting, workforce training, new IPC leadership in the U.S. and Canada, the effects of tariffs, CFX standards, and much more—all designed to provide perspective as you move through the cloud bank of today's shifting economic market.
Intelligent Test and Inspection
Are you ready to explore the cutting-edge advancements shaping the electronics manufacturing industry? The May 2025 issue of SMT007 Magazine is packed with insights, innovations, and expert perspectives that you won’t want to miss.
- Articles
- Columns
- Links
- Media kit
||| MENU - smt007 Magazine
Estimated reading time: 6 minutes
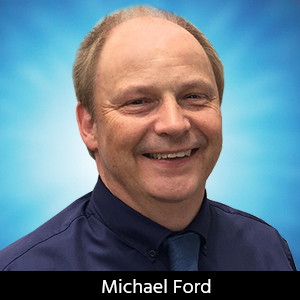
Smart Factory Insights: Hands-off Manufacturing
Assuming perfect manufacturing engineering and reliable process, then product reliability issues are always caused by variation, whether as the result of a simple operational mistake by a person, the wear of a mechanical component on a machine, deviations in material characteristics, or simply a change in the environment. The use of automation has not eliminated causes of unreliability, nor defects, which ironically continues to drive the need for humans to be hands-on, even as part of SMT operations. There is clearly something missing, so cue our digital twin.
With successful manufacturing automation, people are there for their brains, not for their hands. If we were to put a red dot on everything that we touched in manufacturing, whether through necessity, we would quickly have a factory that looked like it had contracted measles. Success can be measured by reducing the number of human touches that need to be made to the product, materials, tools, or machines themselves. This rule applies to assembly itself as well as dealing with exceptions that happen. How then to keep our brains focused while keeping fingers on keyboards and away from danger and still creating world-class process and product reliability?
With each innovation of hardware within manufacturing, we lose first-hand sight of both routine and non-routine events that occur, which include abnormalities and trends that negatively impact reliability and quality. Automation only includes the sensors it needs, assuming other factors are taken care of elsewhere, and unless pushed, typically only shares the minimum of information. When humans were all over the manufacturing line, we were aware of surroundings, seeing issues developing out of the corner of our eyes, that we would remember and address. Without the ability to use our eyes, another form of visibility is needed. We need to gather data. The history of gathering and trusting data from manufacturing has been fraught with challenges—thankfully now being resolved through the increased use of the plug-and-play IPC-CFX standard, which drives IIoT data exchange across the whole shop floor. Of course, data is not visibility; it must be contextualized through the IIoT-based MES layer, which builds event information based on disparate data trails, together with known configuration information, material conditions, work-order perspectives, etc., to create the live digital twin of the manufacturing operation. This visibility is our digital peripheral vision without us interacting directly.
It’s not incorrect to say that the method to improve reliability is to be data-driven, but is very much a simplification. Raw data, proprietary data, and even IPC-CFX are not solutions in themselves, including measurements and inspections. Any data that is gathered must be processed in some way in order to create value. Machine learning famously processes raw machine data measurements to refine and improve the operation. This is incorrect; value comes from the interpretation of results, by another party, such that the determination of whether an escape is really a defect or not, for example the result of an inspection by AOI. Qualification and contextualization bring the value. SMT line closed-loop solutions are another example, where software provides qualification of variation in inspection data coming from one or more machines, then applying corrections and compensation to other machines to keep variation under control. However, it is incorrect to believe that the value of such solutions comes solely from the raw data. Every data point is contextualized by analytic software, based on such things as the dimensions of the PCB, the use of different nozzles for different materials, etc. The type of correction is based on the knowledge of the product, work-order, and materials which do, of course, change. A difference in the material vendor, representing variation potentially in the size, shape, or features of that material, could lead to the closed-loop software making a false call. Holistically, further information needs ultimately to come from MES to understand the complete context of the operation.
These two simple examples illustrate the use of contextualized data to make decisions that impact reliability; in other words, preventing defects from occurring not only during manufacturing, but out into the market. Using trend analysis and refinement in the understanding of the threat that variation represents, a whole slew of hands-on actions is prevented that, in a defect-ridden process, compound quality risk geometrically. There are many other areas where hands-off, data-driven manufacturing ensures that no “out of control” condition is reached to the extent that human action is required. Six Sigma is the leading example of a statistical tool that detects in real-time whether a complex series of data points will remain within control limits, or whether there is a chance that the limits could be exceeded in the near future. Six Sigma is therefore a good engine to be used for “AI-based” monitoring. The problem again is that, as with the case of raw data, the use of Six Sigma is only a means to an end, a tool that needs to be used, with algorithms needed that enable it to be effective for use.
The Active Rules Engine is a great way to think about an AI application that resides within the MES solution, and that is responsible for automatically monitoring a great many contextualized trends, making decisions, and raising alarms, much in the same way as our peripheral vision and thoughts did in the past. Some decisions, such as in the case of SMT line closed-loops, lead to decisions that are executed without the need for any human involvement, becoming more common as we learn how to use our digital-twin visibility more effectively. Some potential decisions, however, need to be referred up to humans, who are now able to manage manufacturing operations much more effectively from their screens, seeing the holistic visibility of the problem, contextualized within the entire operation, rather than having to be physically out on the lines. Such challenges include bottlenecks in the flow of the process, the removal of a suspect material, absence of material or tools, or a potential premature failure of a machine part. Being able to recognize the issue and create a solution avoids the initial quality impact of a defect, as well as avoiding most of the consequences of any failure, all from the comfort of your armchair. Reducing the number of defects found in manufacturing indicates the reduction of market reliability issues.
Now that the contextualized data has taken us so far, there is one more step that we can reliably take to promote hands-off manufacturing. There are likely to be various digital solutions in operation on the shopfloor, which, just like machines, will each do fundamentally different things, and most likely, will come from different suppliers with different core-focused areas of expertise. Take for example, the use of AMR (autonomous mobile robots), a technology that is very useful in executing decisions made by our Active Rules Engine, as well as those escalated to humans. AMR fleet management is dependent on knowing the live status of manufacturing completions and requirements, both routine and exceptional. This can easily be orchestrated through MES in which the Active Rules Engine is based, with the AMR fleet manager driving the detailed specific AMR flow and task management, as they cover material transportation, setup and tear-down of materials and tools, product transfers, and tool management, as well as bringing feeders, nozzles, heads, and stencils, etc., in and out of production for routine maintenance. IIoT-based interoperability between shop-floor solutions is essential in allowing automation to work effectively, with the minimum of manual intervention, no matter what challenges appear, leading potentially to the elusive SMT cleanroom, lights-out operation.
Hands-off manufacturing, by addressing causes and effects of variation in the digital domain, with necessary mechanical activities moved outside of the production area where possible, means that the manufacturing physical and digital twin succeed in levels of reliability and quality that no human nor automated solution could ever achieve unaided.
This column originally appeared in the July 2021 issue of SMT007 Magazine.
More Columns from Smart Factory Insights
Smart Factory Insights: Making Rework a Smart Business OpportunitySmart Factory Insights: The Sustainability Gold Rush
Smart Factory Insights: Today’s Manufacturing Jobs Require a New Skill Set
Smart Factory Insights: Compose Yourself, Mr. Ford
Smart Factory Insights: The Smart Business Case for Local PCB Manufacturing
Smart Factory Insights: Manufacturing Digital Twin—Spanners in the Works
Smart Factory Insights: Machines, People, and AI
Smart Factory Insights: Is Sustainability in Manufacturing a Benefit or Burden?